
Have a little trouble getting to work Wednesday morning? You’re not the only one. Uber and Lyft drivers in cities nationwide went on strike during Wednesday’s rush hour commute, and rallied in places like New York and Atlanta, to protest low pay and other labor concerns. The strike is timed to coincide with Uber’s IPO filing on Friday. The flashiest new tech stock to hit the market, Uber is expected to be valued at more than $80 billion when it goes public at the New York Stock Exchange. Drivers argue that Uber and Lyft profit off the backs of employees, leaving them with low wages and without access to full-time employment benefits despite working long hours. “Wall Street investors are telling Uber and Lyft to cut down on driver income, stop incentives and go faster to driverless cars,” New York Taxi Workers Alliance Executive Director Bhairavi Desai said in a statement. “Uber and Lyft wrote in their S1 filings that they think they pay drivers too much already. With the IPO, Uber’s corporate owners are set to make billions, all while drivers are left in poverty and go bankrupt.” NBC News
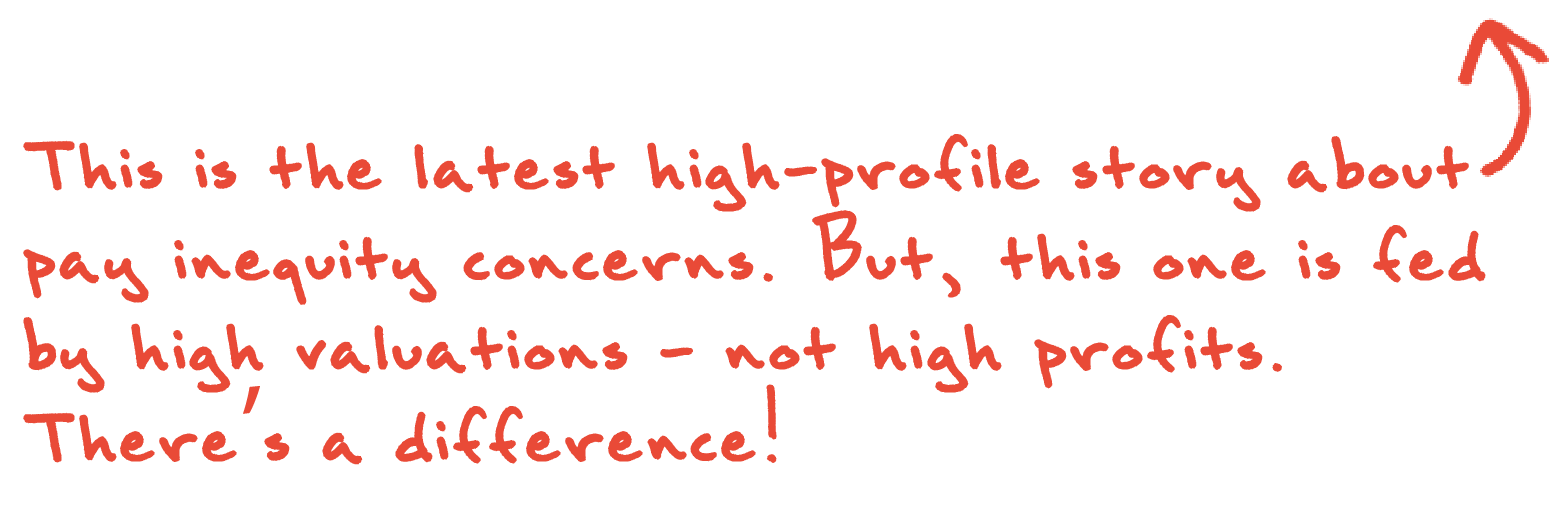

Do hiring algorithms prevent bias, or amplify it? An interesting analysis in Harvard Business Review this week explores that question, which has emerged as a point of tension between the technology’s proponents and its skeptics, but arriving at the answer is more complicated than it appears. Hiring is rarely a single decision, but rather the culmination of a series of smaller, sequential decisions. Many hope that algorithms will help human decision-makers avoid their own prejudices by adding consistency to the hiring process. But algorithms introduce new risks of their own. They can replicate institutional and historical biases, amplifying disadvantages lurking in data points like university attendance or performance evaluation scores. Understanding bias in hiring algorithms and ways to mitigate it requires us to explore how predictive technologies work at each step of the hiring process. Though they commonly share a backbone of machine learning, tools used earlier in the process can be fundamentally different than those used later on. Even tools that appear to perform the same task may rely on completely different types of data, or present predictions in substantially different ways. HBR
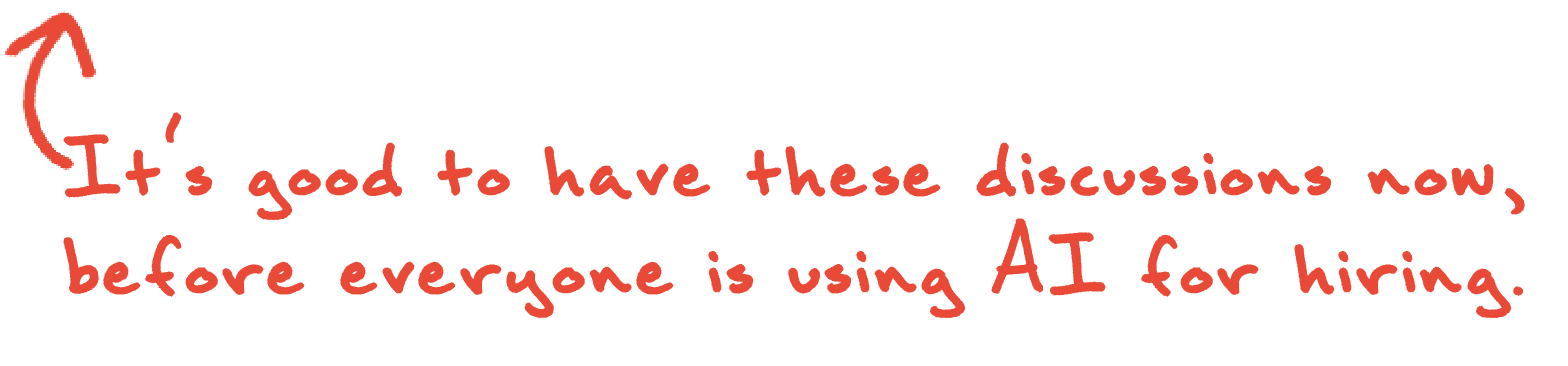

Challenges related to managing religion in the workplace are on the rise, as are religious discrimination claims and monetary settlements. In Cold Call’s podcast this week, Harvard Business School prof Derek van Bever discusses two examples that made their way to the Supreme Court, Abercrombie & Fitch and Masterpiece Cakeshop. We’ve all heard about the cake maker who refused a gay couple’s request for a wedding cake because it violated his religious beliefs — in that case the Supremes sided with him. In Abercrombie, a Muslim woman was denied a sales job because her headscarf violated the company’s extremely strict and WASP-y dress code, called The Look. SCOTUS called it The Discrimination and sided with her. Cold Call Podcast by HBR
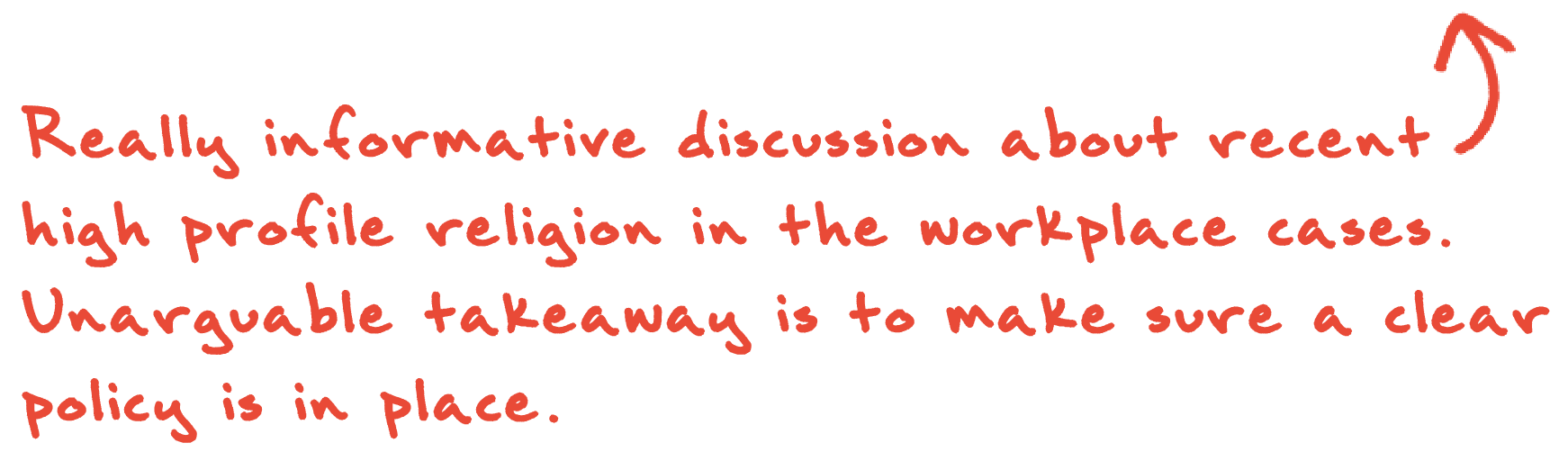

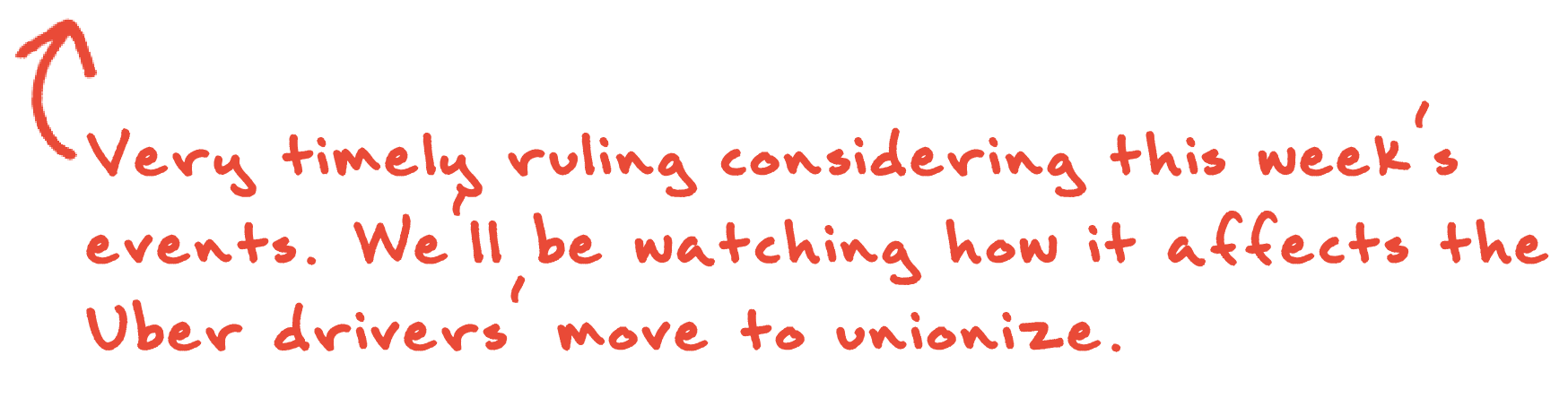

Back in the day, when older people just didn’t get younger people, we called it the generation gap. With five generations in the workplace now, we’ve got gaps all over the place. That’s why there’s so much written about generations at work. Lately, just when we got used to millennials and their man buns, it’s all about the influx of Gen Z, how they differ from their millennial and Gen X elders, and how best to attract, manage, and motivate these kids. That’s all great information, but one teacher in Lowell, Massachusetts, has given HR a practical tool we can use: A dictionary of Gen Z terms so we can actually understand what these kids are saying. No cap! (Translation: I’m serious). One of his students shared it on Twitter and the post went viral, getting more than 500,000 likes. We can only hope some of them were from HR pros. In case you missed it, download it here. WWLP News
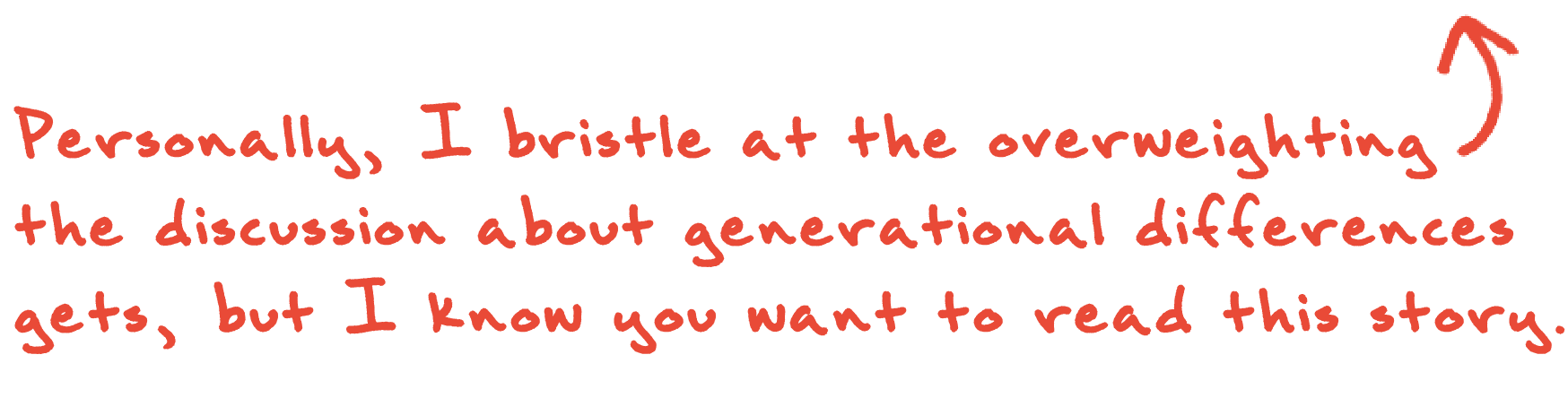